Understanding the White Label AI Voice Agent Landscape
White label AI voice agents represent a transformative opportunity for businesses looking to enter the conversational AI market without building technology from scratch. These ready-to-deploy solutions allow companies to rebrand AI-powered voice systems under their own name, creating new revenue streams with minimal technical investment.
The growth of this sector has been remarkable, with more companies seeking to leverage voice AI technology while maintaining their brand identity. According to recent industry analysis, the white label AI voice agent market is projected to reach significant expansion in the coming years as demand for automated customer service solutions continues to rise.
However, navigating this space requires careful planning and awareness of common pitfalls. Many businesses rush into white labeling without proper understanding of the technology’s limitations, integration requirements, or customer expectations.
The difference between success and failure often comes down to strategic implementation rather than simply acquiring the technology. As studies in AI adoption show, organizations that thoroughly plan their AI implementations achieve substantially better outcomes than those taking a less structured approach.
Poor Understanding of Target Market Needs
One of the most critical mistakes in deploying white label AI voice agents is failing to understand the specific requirements of your target market. Many businesses treat AI voice technology as a one-size-fits-all solution, overlooking the unique needs of their customer base.
Different industries have distinct communication patterns, terminology, and customer service expectations. A voice agent designed for healthcare will require different capabilities than one created for retail or financial services. Without thorough market research, your white label solution may miss the mark entirely.
This mistake commonly manifests when businesses focus excessively on technological features rather than customer problems that need solving. As explored in our article on AI for sales, the most effective AI implementations begin with clear use cases rather than technological capabilities.
Successful white label vendors invest time in understanding industry-specific requirements before customization begins. This includes analyzing common customer inquiries, industry compliance requirements, and communication preferences unique to each vertical market.
Companies should conduct detailed customer journey mapping to identify specific touchpoints where AI voice agents can add the most value, rather than attempting to replace entire communication channels at once.
Insufficient Customization and Branding
Another prevalent mistake is underestimating the importance of thorough customization in white label voice agent solutions. The term "white label" implies making the product truly your own, but many businesses settle for minimal adjustments that leave the underlying generic nature of the solution visible to users.
Effective customization goes far beyond simply adding a logo and changing color schemes. It requires aligning the voice agent’s personality, language patterns, and problem-solving approaches with your brand identity. Customers should experience a seamless extension of your brand when interacting with your AI voice agent.
Voice characteristics, including tone, pacing, and even accent choices, significantly impact how users perceive your brand through AI interactions. As detailed in our guide on AI voice assistants for FAQ handling, voice selection is a critical component of creating an authentic brand experience.
Many businesses neglect to customize the conversation flows sufficiently, resulting in interactions that feel rigid and impersonal. The goal should be creating natural conversational pathways that reflect how your human representatives would handle similar situations.
Successful implementation requires investing in professional voice talent or high-quality text-to-speech technology, as explored in our definitive guide to voice synthesis technology, to ensure the voice agent represents your brand standards appropriately.
Neglecting Integration Capabilities
A major pitfall in white label AI voice agent implementation is underestimating the importance of seamless integration with existing business systems. Many organizations discover too late that their chosen solution operates as an isolated system, disconnected from their critical operational infrastructure.
Effective AI voice agents need to interface with numerous systems: CRM platforms, customer support ticketing systems, knowledge bases, e-commerce platforms, and internal databases. Without proper integration, agents can’t access the information needed to deliver meaningful assistance, resulting in frustrated customers and inefficient operations.
Before selecting a white label solution, businesses should thoroughly evaluate its API capabilities, pre-built connectors, and integration flexibility. As highlighted in our article on AI phone agents, the ability to connect with existing business systems dramatically impacts an AI agent’s effectiveness.
Integration planning should also consider future scalability needs. A solution that meets your current integration requirements might become inadequate as your business grows or adopts new systems. Look for white label providers that prioritize interoperability and maintain robust development roadmaps.
The technical debt accumulated from poor integration decisions can eventually outweigh the initial benefits of implementing an AI voice agent, requiring costly and time-consuming remediation efforts later.
Ignoring Compliance and Security Requirements
Perhaps the most dangerous mistake in white label AI voice agent implementation is neglecting compliance and security considerations. Voice agents often handle sensitive customer information and operate under various regulatory frameworks depending on industry and geography.
Many businesses overlook crucial compliance requirements such as GDPR, HIPAA, PCI DSS, or industry-specific regulations when deploying white label voice solutions. This oversight can lead to significant legal liabilities, financial penalties, and reputational damage.
Data security represents another critical concern. White label AI voice agents process and store valuable customer information, making them potential targets for cybersecurity threats. Thorough security assessment should be a prerequisite for any implementation, as explored in our guide to conversational AI.
Businesses must verify that their white label provider implements robust encryption protocols, secure data handling practices, and regular security audits. Documentation of these measures should be readily available to satisfy both internal governance requirements and customer inquiries.
Additionally, transparency about AI use in customer interactions is increasingly important. Many jurisdictions are implementing regulations requiring disclosure when customers interact with automated systems rather than humans.
Inadequate Training Data and Knowledge Base
A fundamental mistake that undermines AI voice agent effectiveness is providing insufficient training data and knowledge resources. Many businesses dramatically underestimate the volume and variety of information needed to create a truly helpful AI voice agent.
White label solutions typically come with basic conversational capabilities, but creating an agent that can competently address your specific business context requires extensive customization. This includes feeding the system with industry terminology, product information, common customer questions, and appropriate responses.
Organizations frequently fail to develop comprehensive knowledge bases that cover the full range of potential customer inquiries. As discussed in our article on creating your LLM, the quality of an AI system directly correlates with the quality of its training data.
Another common oversight is failing to update the knowledge base regularly as products, services, policies, or market conditions change. Static information quickly becomes outdated, leading to inaccurate responses that damage customer trust.
Successful implementation requires establishing systematic processes for knowledge management, including regular content reviews, updates, and expansions based on actual customer interactions. This requires dedicated resources and should be budgeted as an ongoing operational expense rather than a one-time implementation cost.
Unrealistic Expectations and Scope Creep
Setting unrealistic expectations represents another critical failure point in white label AI voice agent projects. Many businesses overestimate what current AI technology can accomplish or expand project scope beyond reasonable boundaries, leading to implementation failures and disappointed stakeholders.
Organizations often expect voice agents to handle complex, nuanced customer scenarios that require human judgment or emotional intelligence. While AI capabilities are advancing rapidly, as explored in our analysis of AI calling agents for real estate, they still have significant limitations when dealing with complex or emotionally charged situations.
Project scope frequently expands during implementation as stakeholders add "just one more feature" without considering the cumulative impact on project complexity, timeline, and resource requirements. This scope creep can transform manageable projects into unwieldy initiatives that fail to deliver core functionality effectively.
Setting realistic goals requires honest assessment of AI capabilities, understanding true customer needs, and prioritizing features that deliver tangible business value. Starting with limited, high-impact use cases allows organizations to achieve early wins while building capability for more ambitious applications.
Communication with internal stakeholders about AI limitations is equally important. Setting appropriate expectations about what the system can and cannot do helps prevent disappointment and supports more effective human-AI collaboration.
Poor Transition Management
Inadequate transition planning when implementing white label AI voice agents often leads to internal resistance and operational disruptions. Many organizations underestimate the human factors involved in successful AI adoption.
Customer service representatives and sales teams may view AI voice agents as threats to their jobs rather than tools to enhance their capabilities. Without proper change management, this perception can lead to active or passive resistance that undermines implementation success.
Effective transition requires comprehensive communication about how AI voice agents will augment human capabilities rather than replace them. As detailed in our guide on using AI for sales, the most successful implementations position AI as a collaborative tool that handles routine tasks while allowing humans to focus on higher-value activities.
Training programs for staff who will work alongside AI systems are essential yet often overlooked. Employees need to understand how to monitor AI performance, when to intervene in conversations, and how to provide feedback that improves system capabilities over time.
Additionally, organizations frequently fail to establish clear escalation pathways for situations the AI cannot handle. Without well-defined processes for transitioning from automated to human support, customer experiences can deteriorate rapidly when the AI reaches its capability limits.
Inadequate Testing and Quality Assurance
Another critical mistake in white label AI voice agent deployment is insufficient testing and quality assurance. Many organizations rush implementation to achieve quick returns on investment, neglecting thorough validation of the system’s capabilities under various conditions.
Effective testing must cover numerous dimensions: technical functionality, conversational ability, integration reliability, security measures, compliance adherence, and performance under varying load conditions. Each aspect requires specific testing methodologies and success criteria.
Conversational testing is particularly challenging as it must evaluate the agent’s ability to understand diverse phrasings, accents, and communication styles. As explored in our assessment of German AI voices, language variations can significantly impact voice agent effectiveness.
User acceptance testing with actual customers provides invaluable insights that internal testing cannot replicate. However, many organizations skip this step, leading to unpleasant surprises when the system encounters real-world scenarios.
Quality assurance should extend beyond pre-launch testing to include ongoing monitoring and evaluation. Systematic review of customer interactions, success rates, and satisfaction metrics helps identify improvement opportunities and emerging issues before they become significant problems.
Lack of Continuous Learning and Improvement Processes
Treating white label AI voice agent implementation as a one-time project rather than an ongoing program represents a fundamental error. Many businesses fail to establish processes for continuous learning and improvement, severely limiting their solution’s long-term effectiveness.
AI voice agents should improve over time through analysis of actual customer interactions. This requires establishing feedback loops, regular performance reviews, and systematic implementation of improvements based on identified patterns and issues.
Organizations often neglect to allocate resources for ongoing optimization after initial implementation. As detailed in our article on AI appointment booking bots, maintaining effective AI systems requires dedicated attention rather than occasional maintenance.
Human oversight remains essential for identifying subtle issues that automated monitoring might miss. This includes tone problems, cultural misunderstandings, or emerging customer needs that weren’t anticipated during initial design.
Successful implementations treat the first deployment as a foundation for continuous improvement rather than a finished product. This mindset supports regular feature enhancements, conversation refinements, and knowledge base expansions that keep the solution relevant and effective as customer needs evolve.
Ignoring Analytics and Performance Measurement
A significant mistake that limits AI voice agent effectiveness is failing to implement comprehensive analytics and performance measurement systems. Many businesses deploy white label solutions without establishing clear metrics for success or tools to monitor ongoing performance.
Effective performance measurement requires defining specific key performance indicators (KPIs) that align with business objectives. These might include resolution rates, customer satisfaction scores, handling times, escalation frequencies, or conversion rates for sales-focused applications.
Organizations should establish baseline metrics before implementation to enable meaningful comparisons after deployment. Without this foundation, it becomes difficult to quantify the actual business impact of the AI voice agent.
Analytics should extend beyond operational metrics to include conversation analysis that identifies common customer issues, emerging trends, and potential optimization opportunities. These insights can inform product development, marketing strategies, and customer service improvements beyond the AI system itself.
As explored in our guide on reducing cart abandonment with AI phone agents, connecting AI performance to specific business outcomes provides powerful justification for continued investment in the technology.
Insufficient Resources for Maintenance and Support
Underestimating the resources required for ongoing maintenance and support represents another common pitfall in white label AI voice agent implementations. Many organizations allocate adequate resources for initial deployment but fail to budget for long-term operational needs.
Effective AI voice agents require regular updates to their knowledge bases, conversation flows, and integration connections as business conditions evolve. Without dedicated resources for these activities, the system’s effectiveness deteriorates over time as information becomes outdated or incomplete.
Technical support requirements often exceed expectations, particularly during early deployment phases. Organizations must be prepared to address integration issues, performance concerns, and unexpected behaviors that emerge as the system encounters diverse real-world scenarios.
Vendor management represents another overlooked resource requirement. White label relationships require ongoing coordination with technology providers regarding upgrades, feature requests, and issue resolution. As discussed in our article on AI for resellers, maintaining productive vendor partnerships requires dedicated attention.
Successful implementations build resource planning around the entire solution lifecycle rather than focusing exclusively on initial deployment. This includes budgeting for regular updates, technical support, vendor management, and periodic technical refreshes as the underlying technology evolves.
Poor Communication of AI Limitations to Customers
Failing to clearly communicate AI voice agent capabilities and limitations to customers leads to frustration and disappointment. Many businesses oversell what their AI solutions can do, creating unrealistic expectations that harm customer relationships when the reality falls short.
Transparency about AI use is increasingly important to consumers. Organizations should clearly indicate when customers are interacting with automated systems rather than attempting to disguise them as human agents, which can damage trust when the deception becomes apparent.
Effective communication includes setting appropriate expectations about what the AI can handle and providing clear pathways to human assistance when needed. As explored in our examination of virtual call powers, the most successful implementations create seamless transitions between automated and human support.
Organizations should develop scripts that honestly acknowledge the AI’s limitations when appropriate. Rather than pretending the system can handle every scenario, these acknowledgments help manage customer expectations and reduce frustration when human intervention becomes necessary.
Customer education about effective interaction with AI systems can significantly improve satisfaction. Simple guidance about speaking clearly, using straightforward language, and asking one question at a time can dramatically improve resolution rates and customer experiences.
Overlooking Voice Quality and Natural Language Processing Capabilities
A technical mistake that significantly impacts user experience is underestimating the importance of voice quality and natural language processing (NLP) capabilities. Many businesses select white label solutions based primarily on feature lists and pricing without adequately evaluating these fundamental components.
Voice quality dramatically influences how customers perceive AI agents. Synthetic voices with unnatural cadences, inappropriate emotional tones, or pronunciation errors create negative impressions regardless of the system’s functional capabilities. As examined in our ElevenLabs review, voice technology quality varies significantly between providers.
NLP capabilities determine how effectively the system understands customer inquiries, particularly when they include industry jargon, complex questions, or conversational interruptions. Limited NLP implementation results in frequent misunderstandings that frustrate users and reduce resolution rates.
Multilingual capabilities represent another frequently overlooked requirement. Organizations serving diverse customer populations need solutions that can effectively handle multiple languages rather than forcing non-native speakers to struggle with limited language options.
Thorough evaluation should include testing with actual customer conversations rather than simplified demonstration scenarios. This reveals how the system handles real-world complexity, including background noise, speech variations, and the meandering nature of actual customer inquiries.
Failure to Consider Scalability Requirements
Neglecting to evaluate scalability requirements represents a strategic error that creates significant problems as business needs evolve. Many organizations select white label AI voice agent solutions based on current requirements without considering future growth scenarios.
Scalability encompasses multiple dimensions: call volume handling, knowledge base expansion, language support, integration capabilities, and administrative management. Limitations in any of these areas can create bottlenecks that prevent the system from growing alongside the business.
Technical architecture significantly impacts scalability potential. Cloud-based solutions typically offer greater flexibility than on-premises implementations, but vary considerably in their ability to handle rapid growth or usage spikes. As detailed in our SIP trunking guide, underlying communication infrastructure plays a crucial role in system scalability.
Cost models represent another scalability consideration. Some solutions become prohibitively expensive at higher volumes due to per-transaction pricing, while others offer more favorable economics for large-scale deployments.
Organizations should develop realistic growth projections and evaluate potential solutions against these scenarios rather than focusing exclusively on immediate requirements. This forward-looking approach helps avoid costly platform migrations when initial solutions prove inadequate for evolving needs.
Neglecting Multi-Channel Integration
A strategic oversight that limits AI voice agent effectiveness is failing to integrate voice capabilities with other communication channels. Many businesses implement voice AI as a standalone solution rather than part of a cohesive multi-channel customer experience strategy.
Modern consumers expect seamless transitions between communication channels, including voice, chat, email, mobile apps, and web interfaces. They become frustrated when forced to repeat information or restart processes when moving between channels. As explored in our article on omnichannel strategies, integrated experiences significantly improve customer satisfaction.
Voice agent implementations should coordinate with existing digital channels to maintain conversation context and customer history across interactions. This requires thoughtful integration planning and data sharing between systems that many organizations neglect.
Channel preferences vary significantly between customer segments and use cases. Effective strategies recognize these variations and offer appropriate options rather than forcing all interactions into a single channel, regardless of suitability.
Organizations should develop comprehensive journey maps that identify how and when customers might transition between channels during complex interactions. This planning helps create smooth handoffs that maintain context rather than disjointed experiences that frustrate users.
Transforming Your Business with White Label AI Voice Agents
After exploring the common mistakes to avoid, it’s clear that implementing white label AI voice agents requires strategic planning, ongoing commitment, and realistic expectations. When properly executed, these solutions offer tremendous business value through improved customer experiences, operational efficiency, and competitive differentiation.
The most successful implementations begin with clear business objectives rather than technology fascination. They align AI capabilities with specific customer needs, integrate seamlessly with existing systems, and provide transparent pathways to human assistance when needed.
Voice agent technology continues advancing rapidly, with improvements in natural language understanding, emotional intelligence, and conversational capabilities. Organizations that establish solid foundations now position themselves to leverage these advancements as they emerge.
As illustrated across our discussions of AI phone calls, AI cold calls, and AI call centers, the potential applications continue expanding across industries and use cases. From appointment scheduling to customer support, sales outreach to service delivery, AI voice agents are transforming how businesses communicate.
If you’re considering implementing white label AI voice agents in your business, I encourage you to explore the comprehensive capabilities available through Callin.io. Our platform enables you to deploy sophisticated AI phone agents that handle both inbound and outbound communications autonomously. With natural conversational abilities, our AI agents can schedule appointments, answer common questions, and even close sales by interacting naturally with your customers.
Callin.io offers a free account with an intuitive interface for configuring your AI agent, including test calls and access to the task dashboard for monitoring interactions. For advanced features like Google Calendar integration and built-in CRM functionality, subscription plans start at just $30 per month. Learn more by visiting Callin.io today.
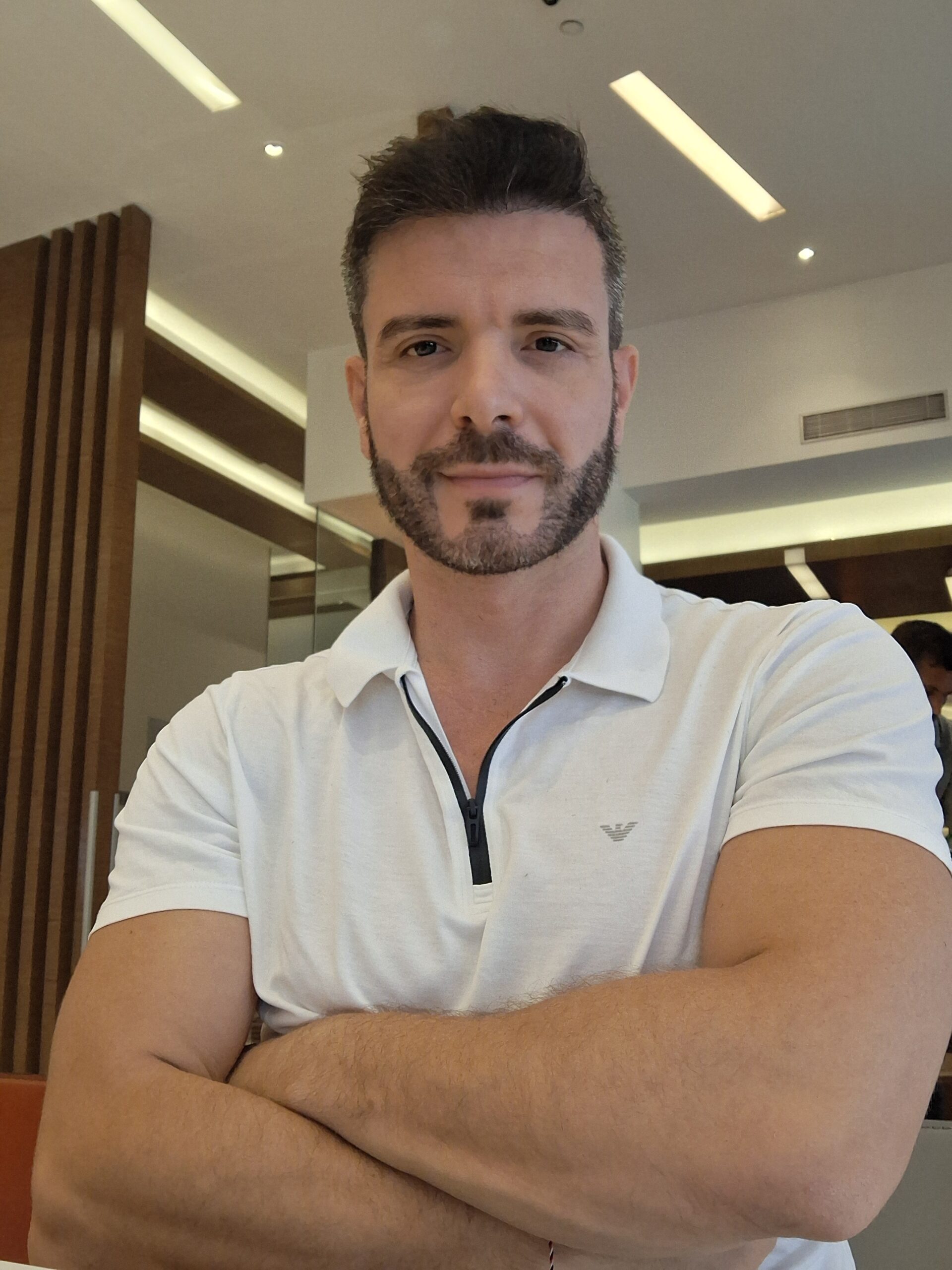
Helping businesses grow faster with AI. π At Callin.io, we make it easy for companies close more deals, engage customers more effectively, and scale their growth with smart AI voice assistants. Ready to transform your business with AI? π Β Letβs talk!
Vincenzo Piccolo
Chief Executive Officer and Co Founder